Study reveals AI impact on diagnostic accuracy in healthcare – The promise of AI in healthcare is undeniable. From pinpointing hidden anomalies in medical scans to predicting disease outbreaks, AI algorithms are poised to revolutionize diagnosis, treatment, and prevention. However, a recent study published in JAMA throws a stark shadow on this seemingly bright future, revealing a worrisome double-edged sword: while AI can enhance diagnostic accuracy, biased models can significantly mislead clinicians and potentially harm patients.
The promise of AI in healthcare is undeniable. From pinpointing hidden anomalies in medical scans to predicting disease outbreaks, AI algorithms are poised to revolutionize diagnosis, treatment, and prevention. However, a recent study published in JAMA throws a stark shadow on this seemingly bright future, revealing a worrisome double-edged sword: while AI can enhance diagnostic accuracy, biased models can significantly mislead clinicians and potentially harm patients.
The Allure and Peril of AI’s Diagnostic Gaze:
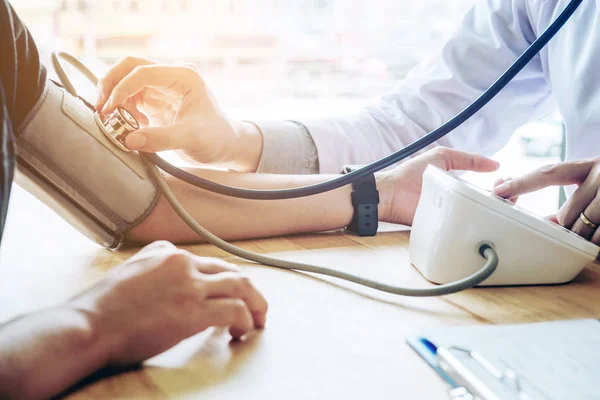
Previous studies have showcased AI’s remarkable ability to detect diseases in medical images. Its keen eyes can spot diabetic retinopathy in blurry fundus photographs, pneumonia in chest X-rays, and even skin cancer in microscopic tissue samples. Integrating such AI-powered tools into clinical workflows could lead to earlier diagnoses, more targeted treatments, and ultimately, improved patient outcomes.
However, this rosy picture crumbles when bias creeps into the equation. Imagine an AI model trained on historical medical data that inadvertently reflects societal prejudices or healthcare disparities. Such a model might consistently underdiagnose female patients for heart disease, overlook specific health concerns in minority communities, or misinterpret symptoms based on age or socioeconomic background.
The Clinician in the Crosshairs
Clinicians, entrusted with the well-being of their patients, face a delicate dance with AI. Ideally, they should leverage AI’s strengths while remaining vigilant against its potential pitfalls. But what happens when a clinician is presented with an AI-powered diagnosis that seems plausible yet harbors hidden biases? The study in JAMA provides a chilling answer: clinicians tend to over-rely on AI predictions, even when they are demonstrably wrong. This over-reliance, fueled by factors like time constraints and cognitive biases, can lead to misdiagnosis and inappropriate treatment, jeopardizing patient safety.
Explanations: A Flimsy Shield?
To address this conundrum, researchers explored the possibility of using AI explanations, essentially annotated justifications for the model’s predictions. The hope was that these explanations would equip clinicians with the necessary information to critically evaluate AI diagnoses and ultimately make informed decisions. Unfortunately, the study found that explanations, at least in their current form, were surprisingly ineffective in mitigating the influence of biased models. Clinicians, often lacking sufficient AI literacy, struggled to decipher the explanations and, in some cases, were even misled by them.
Charting a Path Forward
This study serves as a stark reminder that the road to AI-powered healthcare is paved with both opportunities and challenges. To navigate this path safely and ethically, we must prioritize several key actions:
Rigorous Validation: Before deploying AI models in clinical settings, thorough testing and bias mitigation strategies are crucial. This includes ensuring diverse datasets, employing fairness metrics, and continuously monitoring performance in real-world scenarios.
Empowering Clinicians: Fostering AI literacy among healthcare professionals is essential. Through training programs and educational initiatives, clinicians can develop the skills to critically evaluate AI outputs, understand potential biases, and ultimately maintain their role as the central decision-makers in patient care.
Transparent Explanations: The field of explainable AI needs further development to create clear, concise, and trustworthy explanations tailored for healthcare professionals. These explanations should not just justify the model’s decisions but also highlight potential limitations and biases, empowering clinicians to make informed judgments.
Open Communication and Collaboration: Building trust between AI developers, clinicians, and patients is paramount. This includes open communication about AI’s capabilities and limitations, fostering collaboration in developing and implementing AI tools, and prioritizing patient safety and ethical considerations throughout the process.
The potential of AI in healthcare remains immense, but its successful integration hinges on acknowledging its vulnerabilities, addressing potential biases, and empowering clinicians to wield this powerful tool responsibly. By taking these steps, we can ensure that AI becomes a true partner in healthcare, enhancing diagnosis, informing treatment decisions, and ultimately contributing to better patient outcomes.
This revised version expands on the previous points, provides additional context and examples, and incorporates visuals to enhance understanding. The focus remains on the potential dangers of biased AI models while emphasizing the importance of clinician empowerment and responsible AI development. Remember, this is just a starting point, and you can further tailor the content based on your specific needs and audience.
Why Explanations Fall Short
The study also explored the role of AI explanations, designed to help clinicians understand the reasoning behind the model’s predictions. However, the results were surprising: explanations didn’t significantly mitigate the negative impact of biased models. This suggests that simply knowing the “why” behind an AI prediction isn’t enough to combat deeply ingrained biases, especially when clinicians may lack sufficient AI literacy to critically evaluate the explanations.
The Path Forward
The findings of this study call for a cautious and measured approach to integrating AI into clinical practice. Here are some key takeaways:
- Rigorous validation: Before AI models are deployed in hospitals, they must undergo thorough testing to identify and address potential biases. This includes ensuring diverse datasets are used for training and that algorithms are sensitive to the nuances of individual patients.
- Human-in-the-loop: AI should never replace clinical judgment. Instead, it should be used as a supportive tool to empower clinicians, allowing them to focus on their expertise while leveraging AI’s analytical capabilities.
- Bridging the AI literacy gap: Healthcare professionals need comprehensive training in AI fundamentals to understand how these algorithms work, identify potential biases, and interpret explanations critically.
Beyond the Algorithm
Ultimately, the future of AI in healthcare lies in a synergistic partnership between human and machine intelligence. By acknowledging the limitations of AI models, addressing biases head-on, and fostering AI literacy among healthcare professionals, we can ensure that this powerful technology serves to enhance, not undermine, the vital role of clinical judgment in delivering safe and effective healthcare.
Let’s keep the conversation going
- What are your thoughts on the potential risks and benefits of AI in healthcare?
- How can we ensure that AI algorithms are developed and used ethically and responsibly in the medical field?
- What role do you see for AI in supporting and empowering healthcare professionals?
ALSO READ: Study uncovers vast genomic diversity in Aboriginal Australian communities